TITLE:
Twitter Data Pre-Processing and Detection of Fake Reviews
AUTHORS:
Shrinivas Biradar, Dr. G T Raju, Divakar K M
DOI: 10.5110/77. 1097 Page: 72-86 Vol: 19 Issue: 01 Year: 2024
ABSTRACT
Over last few decades, Twitter has grown enormously. It is utilized now by a million people who disseminate knowledge about their lives and feelings. However, to process and analyze this information, evaluation of sentiment and theme modeling methods are used by the applications. The preliminary processing procedures necessary to derive characteristics using Twitter data are detailed in this study. The technique enables an improvement in sentiment estimation efficiency. In the past couple of decades, sentiment analysis of Tweets has advanced significantly by conducting many experiments in this area of expertise. Several machine-learning algorithms have been employed over the years and anticipate being employed in the future for sentiment analysis in the upcoming days to accomplish further accurate results. Nowadays people possess knowledge about platforms a place where people could convey opinions freely, hence this provides a chance for sentimental analysis could be carried through and the findings utilized for various goals since it may provide insight into a person’s intense condition. There are three main techniques employed in Sentiment Analysis: 1. Machine learning-based 2. Sentiment lexicon-based 3. Hybrid approach. In this study, we propose an approach that involves extracting attributes once an initial preprocessing of tweets. Furthermore, we utilize Logistic Regression, Naïve Bayes, and Support Vector Machine (SVM) Classifiers to detect fraudulent reviews. The rise of online platforms and the growing influence of user-generated content have prompted a rise in the predominance of fake reviews. Fake reviews can be harmful to businesses and consumers alike, as they mislead potential customers and mislead market dynamics. Detecting fake reviews has become a critical task in maintaining the integrity of online review systems. Nevertheless, fake review detection poses numerous challenges. Confrontational attacks by individuals or groups trying to avoid detection classifiers require continuous refinement and adaptation of detection techniques. In this paper, the main objective is to highlight the significance of data pre-processing techniques and show how it could benefit to enhance the accuracy. In this paper, we additionally elucidate the factors contributing to the enhanced accuracy through a meticulous examination of each approach.
Keywords:
Sentiment Analysis, Machine Learning, Logistic Regression, Naïve Bayes, SVM, Twitter, Pre-Processing
Received: 26 December 2023
Accepted: 06 January 2024
Published: 13 January 2024
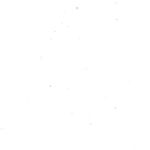